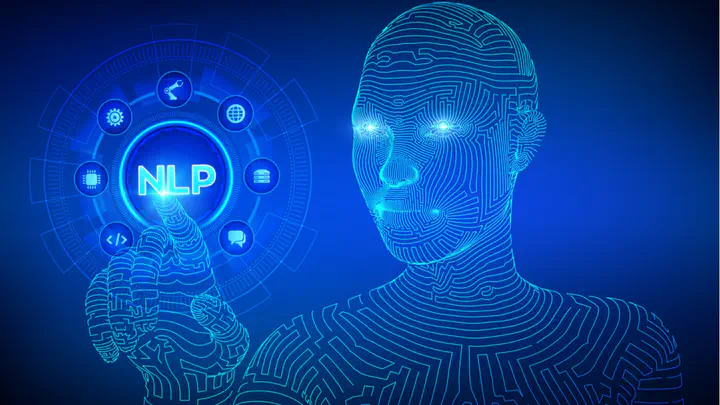
This work aims to address the problem of concept-based explainability in natural language understanding tasks. Concept-based interpretability methods are important to helping users understand model predictions in human-level intuitive concepts. While prior works in concept-based explainabilty have largely focused on the vision domain, we explore challenges in adapting such methods to natural langauge by building from recent works in this area and applying concept-based explainability methods to a sentiment classification task. We compare the concept-based interpretable methods to black-box model predictions and find that performance on the task is comparable, however the coherency of discovered concepts in the interpretable model suffers from lack of coherency.